Web apps need to be quick by reducing latency while serving client requests. In all applications persistent data is on a database on disk (some use solid state too) and the web app on server accesses it. While a few concurrent connections will be easily handled by issuing database requests, when it comes to thousands or millions of requests this approach will fail fast. Scaling up by utilizing powerful hardware is a solution which can buy time until the next growth level of client requests. Even on a scaled up hardware there is room to squeeze more requests through if data caching is part of the design. Accessing data from disk / another server on the network is slower than accessing it from the memory. If the round trip can be avoided this enables fewer comparatively low cost hardware to easily handle considerable loads without increasing latency. A profiling of response times is on the next post. Once server side things like what is the app's actions, when it acts, how fast & efficiently are thought out, the data model can be designed for maximum throughput too.
Here we look at horizontally deploying multiple application servers and a single back end database which handles these app servers' data requests. These app servers are load balanced in round robin method for requests from the external world. Each request to a webapp is given to a different app server. Sticky sessions can also be enabled but that won't be round robin'ed. The objective of round robin is to decrease latency caused by the wait if it was only one server. However, in this scenario the database handles all the app servers. If not addressed this becomes a contention easily thus taking everything to square one. On closer analysis of most web apps/sites/services it would be possible to avoid unnecessary database calls with caching. (The next level to improve performance would be database sharding) What this helps is saving the cost of a network/disk call. Imagine having a good pizza ready on the kitchen table rather than you having call Dominos' or Pizza hut's home delivery. In the case you had to call home delivery, ask for pizzas and keep some ready in your refrigerator just in case someone suddenly gets the urge thus saving you and them the wait next time. Pizzas become stale, so do data. There has to be precise cache invalidation when the database record is updated. The point is that you might have to hit the database but it is not needed every time a request is made.
Software:
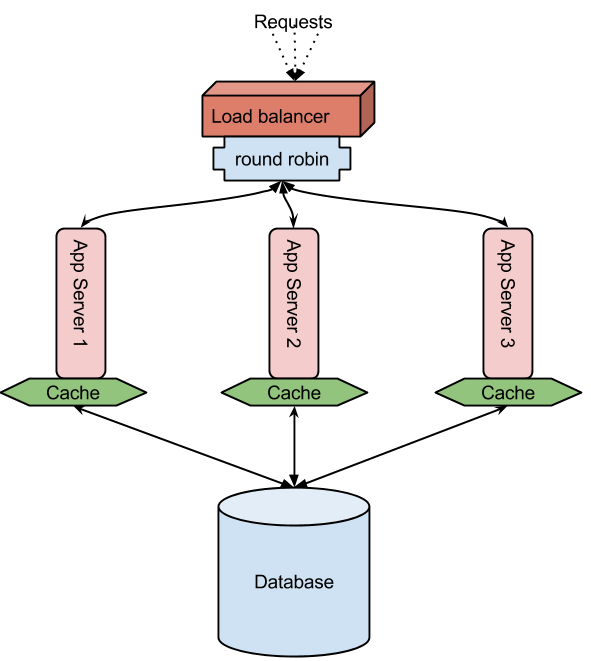
Web app: developed on Django framework.
Webserver: Apache2 with mod_wsgi to host python webapp
Caching: Memcached
Database: Postgresql
Load balancing: nginx
# application servers: 3
# loadbalancing node: 1
Django code with sample view that uses the cache:
Wireshark trace showing the round robin requests and responses